Composability and the 2025 martech stack
The rise of the decisioning layer
This article includes insights from a conversation between Scott Brinker, chiefmartech.com Editor, and George Khachatryan, OfferFit CEO.
For centuries, a mark of human progress has been specialization. Leonardo Da Vinci, they say, was the last true polymath. As the pace of technological progress accelerates, specialization accelerates with it.
In marketing, the inexorable march of specialization can be a mixed blessing. According to Scott Brinker’s 2024 State of Martech Report, the 2024 landscape is made up of a staggering 14,106 products. “We are not a consolidated industry,” explains Scott. “We are a heterogeneous, diverse industry, and with AI we seem to be getting even more diversity and emerging innovation.”
The composable martech stack
The only way that marketers can keep up with – let alone drive value from – this proliferation of tools is through composability. At root, composability is a simple idea – different products in the martech stack should “play nicely” with each other, allowing marketers to pick and choose the product best suited for each need.
In theory, of course, businesses could instead choose one “monolithic” product for all core marketing functions – data, CRM, automation, campaign orchestration, etc. – rather than rely on composability. In practice, the martech ecosystem continues to grow precisely because marketers don’t want a monolith – they want to use the “best in class” product for capability. According to Scott Brinker, 83% of businesses use alternative products in place of built-in features of the central products in their martech stack. Why? 68% of marketers say the reason is better functionality – they want the “best in breed” at each layer in the stack.
The “centers of gravity” of the martech stack
In days of yore – 2010 or so – the archetypal martech stack had the marketing automation platform at its core, with various apps integrated into that central tool.
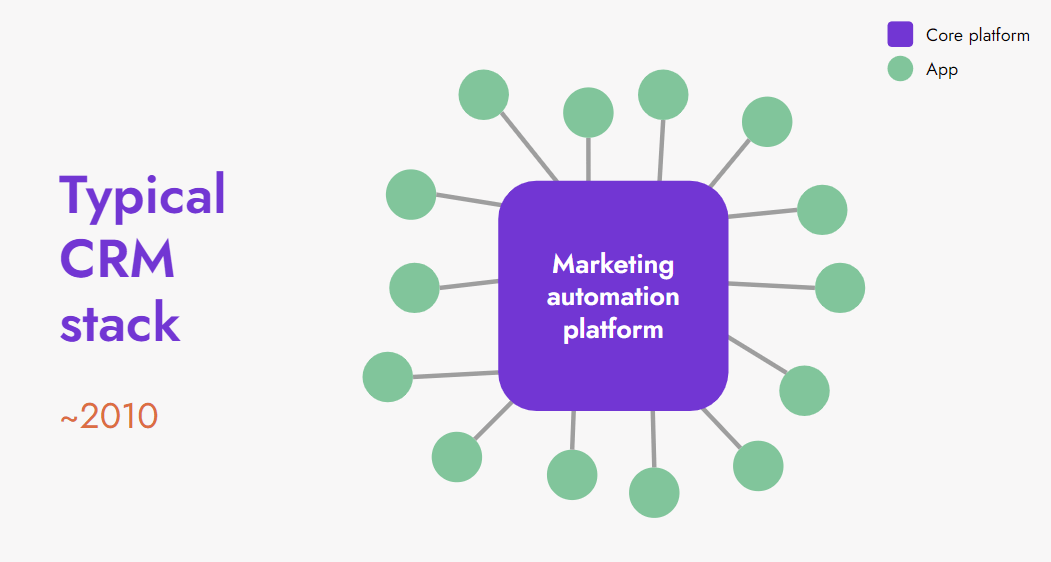
In recent years, as companies have aggregated their customer data, we’ve seen the rise of data warehouses and CDPs. This data layer connects to the marketing automation platform, and both are integrated with other products.
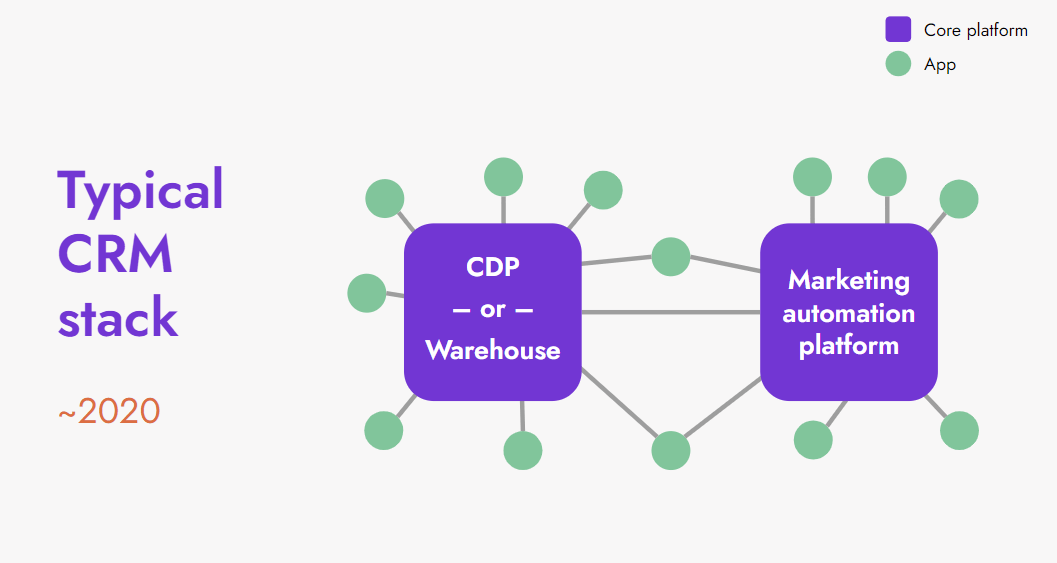
This development created a tension – marketers want the capabilities of a CDP, but duplicating data in both a warehouse and CDP is inefficient and expensive. Hence the rise of “composable” CDPs, which cede the primary “data repository” role to the warehouse, but provide connectivity, identity resolution, and other marketing-facing data capabilities to the marketer.
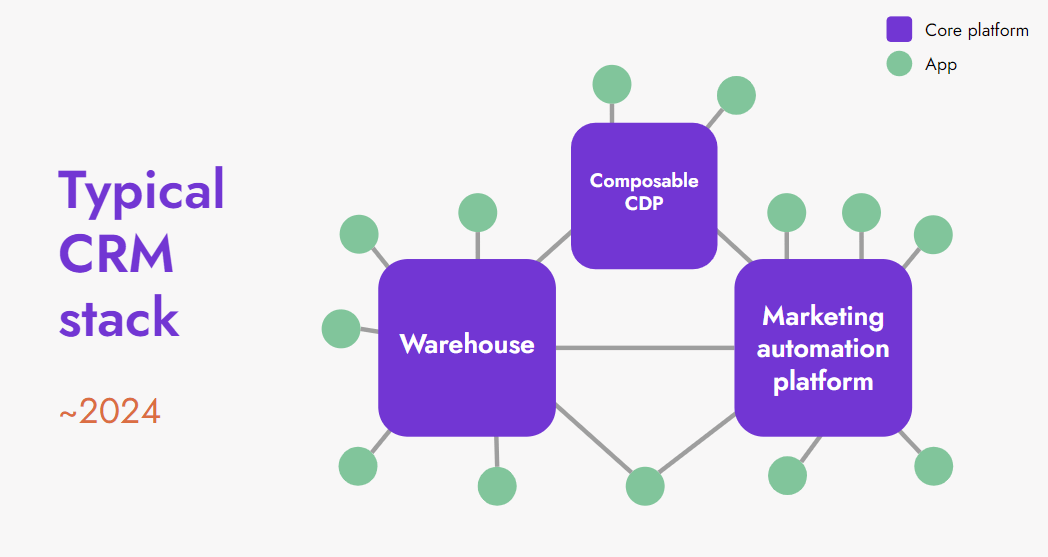
Next best action models and their discontents
Missing from this 2024 stack is a core capability for making decisions about what actions to take for each individual customer. Today, savvy marketers are using tools like next best action models to personalize. Typically, an NBA model combines predictive models and business rules to predict the product or offer to suggest to a customer. Let’s unpack how that works. A traditional NBA model consists of the following steps.
Start with raw customer data in the data warehouse – e.g. purchases, web browsing events, app logins, email clicks.
Use these data to calculate customer features – e.g. purchases in the last 90 days, average value of last 10 purchases, days since last purchase.
Use these features as the input for predictive models – e.g. churn propensity, propensity to repurchase in a given category, customer lifetime value prediction.
(Optional) Segment customers based on these features and models – e.g. create a “high risk” segment of customers with a churn score over 80.
Write business rules for which product to each customer based on the features and models, either for the customer population as a whole, or separate rules for each customer segment.
Assess the effectiveness of these rules through manual A/B or multivariate tests.
Here’s an example of what such a model could look like.
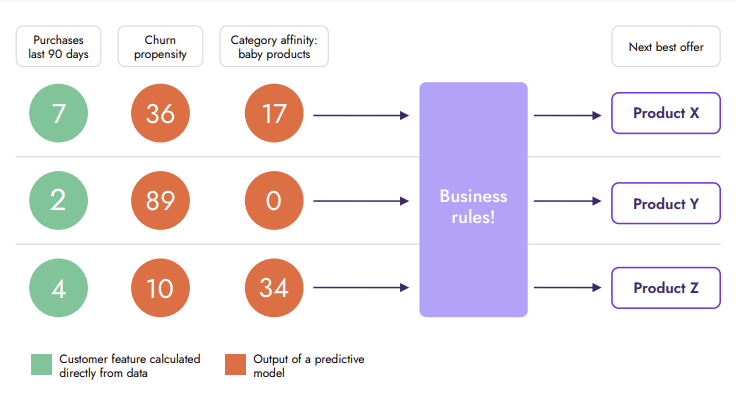
But marketers are realizing that traditional NBA models have significant limitations.
Traditional NBA models are brittle – keeping them up to date as markets and customer behaviors change is both slow and expensive.
Traditional NBA models typically make recommendations for segments, not people. They offer "personalization" which is not very personal.
The only way to know if NBA models are really making optimal decisions is through manual testing – usually A/B testing or multivariate testing by segment.
The “action” the model recommends is usually a product or offer. Traditional NBA models cannot simultaneously optimize all the decisions marketers must make with every customer touchpoint – e.g. message, creative, financial incentive, channel, time, day, frequency – much less handle the interdependencies between these decisions.
The rise of the decisioning layer
Marketers are beginning to realize that using predictive models and business rules to pick “winners for segments” isn’t getting closer to the dream of true 1:1 personalization. A newer technology, AI testing, based on a type of machine learning called reinforcement learning (RL), offers fundamental advantages over the older “next best action” approach.
AI testing models continuously learn and adapt. They are flexible rather than brittle, and automatically adjust to market changes.
AI testing leverages all first-party data about every customer characteristic to make 1:1 decisions about individuals, not segments.
AI testing empirically discovers the best decisions for each customer which maximize the marketer’s KPIs – no need for manual testing.
AI testing can optimize across multiple dimensions simultaneously.
Because 1:1 decisioning is such a critical capability for enterprise marketers, we are seeing a new core platform emerge in the martech stack: the AI decisioning platform, which uses AI testing to make 1:1 decisions for each customer.
The martech stack of 2025 in beyond will have 4 core components.
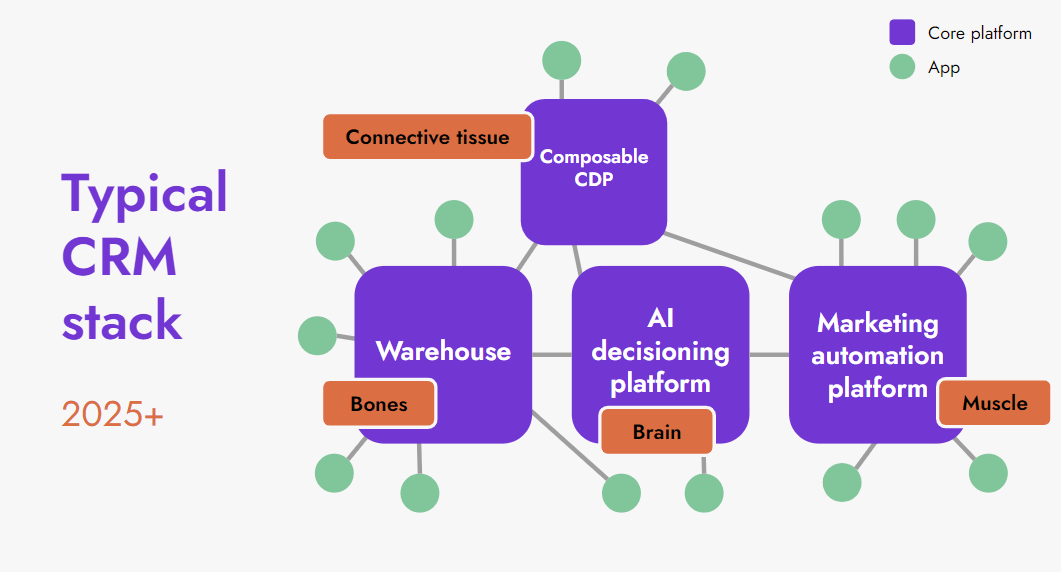
To use perhaps a cheesy analogy: the data warehouse forms the bones of the stack, the composable CDP provides connective tissue, and the automation platform is muscle. But these capabilities, in 2025, will not be enough for marketers to remain competitive.
The martech stack needs a brain.
Nathaniel Rounds writes about AI and machine learning for nontechnical audiences. Before joining OfferFit, he spent 10 years designing and building SaaS products, with an emphasis on educational content and user research. He holds a PhD in mathematics from Stony Brook University.
Experimentation unleashed
Ready to make the leap from A/B to AI?