How the state of the art is shifting in AI-driven personalization
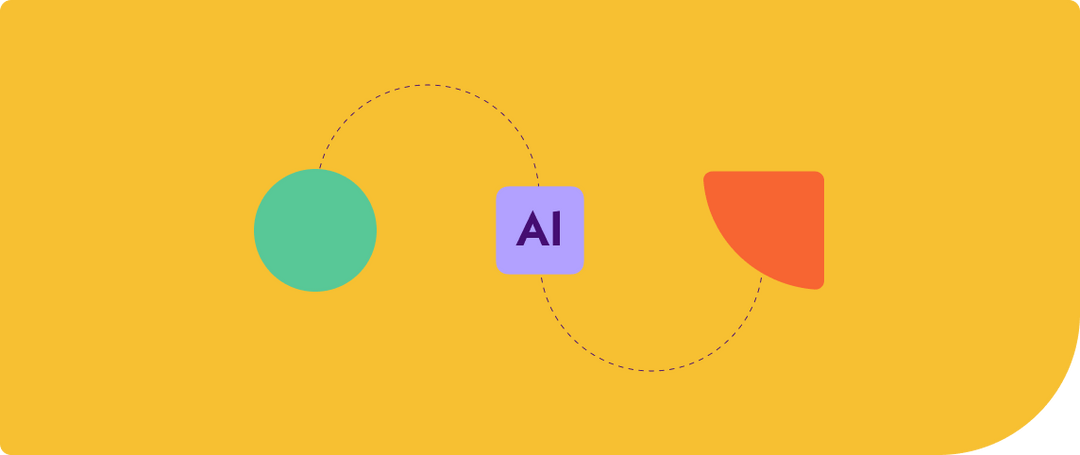
How the state of the art is shifting in AI-driven personalization
Everyone’s talking about AI, but for marketers, that’s old news. Most enterprise marketers have been using AI to personalize for years. Businesses have made tremendous progress in consolidating their data in warehouses or CDPs to build holistic customer views which consolidate engagement, purchase, and marketing events. Centralized data, in turn, has proved fertile ground for machine learning (ML)
models to personalize. But the best practices are changing, and no, not because of chatGPT. Let’s look at how most marketers are using ML today, and how the state of the art is shifting.
The old state of the art
The most common way that marketers are using ML to personalize today is by using predictive models
, and then running multivariate testing by segment
. Let’s unpack how that works. First, marketers build segments by picking the 4-5 customer characteristics that are most important to them. These characteristics could be things like:
Average value of last 10 purchases.
Recency of last purchase.
Average frequency of purchase.
Propensity to repurchase.
Propensity to churn.
Customer lifetime value prediction.
Note that the first three examples above can be calculated directly from data – they don’t require any kind of sophisticated ML. But not all customer characteristics that marketers care about are so easily pinned down.
Marketers often use predictive models
, based on a type of ML called supervised learning
, to estimate, for example, churn or repurchase propensity. A churn model might give every customer a score on a scale from 0 to 100 based on how likely the model thinks the customer is to churn in, for example, the next 3 months. Marketers can segment customers based on churn score, perhaps into quintiles or deciles. Combining the output of various models will give perhaps hundreds of microsegments
. For example, suppose a business picks 4 customer characteristics as the key dimensions by which they wish to segment their customers. The business might split customers into five segments for each – customers with churn scores between 80-100, 60-79, and so forth. Four dimensions, each split into gives segments, gives 5*5*5*5 = 625 microsegments.
Running simultaneous A/B tests on 625 segments is likely infeasible, so businesses typically use multivariate testing
to measure what works best with each segment. From there, marketers author business rules
about which actions to take for each segment – such as what products to offer, what channels to use, or what time of day to send promotions. This method can be effective, and indeed is how many enterprises use ML today to personalize. Perhaps 5 years ago, this method was “state of the art.” But this approach is not without its limitations.
Predictive models are brittle and expensive to maintain
Predictive models are trained and deployed at a point in time, but markets change, and customer behaviors change with them. Suppose a business has a well-built churn prediction model trained on existing data, and business rules based on extensive multivariate tests. Next week, a marketer has an idea for a new offer, creative, or incentive to send to customers at risk for churn. These offers are new – the marketer’s multivariate tests did not include them, and so their business rules have no data about them. And how will these new offers impact customer churn propensity? The churn prediction model wasn’t trained on these offers either. Both the marketer’s business rules, and the underlying predictive models and the customer segments they define, may begin to go awry.
The business now faces a choice: either data scientists must collect training data on the new offers and retrain their model, or marketers must run the risks of bad predictions from an out-of-date model. Simply put, predictive models are brittle. They work well when applied to data very similar to the data they were trained on, but have trouble generalizing to new situations. It does not take a seismic shift like a pandemic or a recession to make the model run off course. Deploying a new offer, or emailing customers with a different frequency, is enough for the models’ predictions to become unreliable. As the collection of models a marketer deploys – churn prediction, repurchase prediction – continues to grow, the effort and expense to keep those models up-to-date will grow with them.
Even if businesses devote time and money to continuously retraining their models, marketers aren’t out of the woods. After all, the marketer ran multivariate tests at a moment in time. The business rules based on the results of these tests are now out of date. The marketer can retest, but that’s more time and expense. Meanwhile, markets and customer behaviors continue to evolve. The business’s choices are not very appealing: devote significant resources to continuously retrain models and rerun tests, stick with outdated segments and rules and hope for the best.
Rules-based personalization isn’t very personal
Even if businesses spare no expense in updating their models and retesting their business rules, there is no escaping a more fundamental problem. “Segments and rules” based personalization is just not very personal! 625 microsegments may sound like a lot, but when a business has thousands or millions of customers, microsegments are a long way from 1:1 personalization.
Marketers want to find the “winners” for each microsegment – the winning message, creative, offer, channel, time of send, and frequency. The results of multivariate tests for 625 segments certainly seem very personal. “For segment X, 10am sends got more conversions than 4pm sends.” Sure sounds like a win! The problem is that each microsegment represents hundreds or thousands of customers, and some of those customers clicked on the 4pm email. If marketers adopt the “winning” option, they are simply letting the majority rule. In an election, getting 51% of the vote means you win. In a marketing campaign, engaging 51% of your customers is probably a losing strategy – 49% of your customers aren’t reading your email. Dividing the customer base into 625 microsegments merely repeats the problem 625 times.
Marketers’ rich first-party data describes hundreds of customer characteristics. Rules built on the results of a handful of predictive models reduces customers to only a few data points – the outputs of each model – letting the depth of first-party data go to waste. Despite the effort businesses are making to continuously update models and rerun tests, the result is personalization that simply isn’t very personal.
The new state of the art: AI testing using reinforcement learning
What marketers really want is to make decisions 1:1 for each individual customer – the right message, with the right offer, sent through the right channel, at the right time, with the right frequency of communication. And of course, they would rather not constantly rerun rests and manually update business rules.
AI testing
, based on a type of ML called reinforcement learning (RL)
, finally makes this dream a reality. The advantages over the old “predictive models, microsegments and rules” approach is fundamental.
RL models
continuously learn and adapt
. They are flexible rather than brittle when the marketer adds new offers or customer behavior changes, and do not require constant tweaking and retraining.RL models leverage
all first-party data
about every customer characteristic to make1:1 decisions
RL models
empirically discover
the best 1:1 decision for each customer – no need for manual business rules, and no need for multivariate tests to see what’s working.
Segments and rules based personalization was great, and still gets good results. But we can no longer say it’s the state of the art.
Ready to learn more about AI testing? Download our whitepaper or schedule a demo with one of our experts.
Nathaniel Rounds writes about AI and machine learning for nontechnical audiences. Before joining OfferFit, he spent 10 years designing and building SaaS products, with an emphasis on educational content and user research. He holds a PhD in mathematics from Stony Brook University.
Experimentation unleashed
Ready to make the leap from A/B to AI?