Why A/B testing is dead
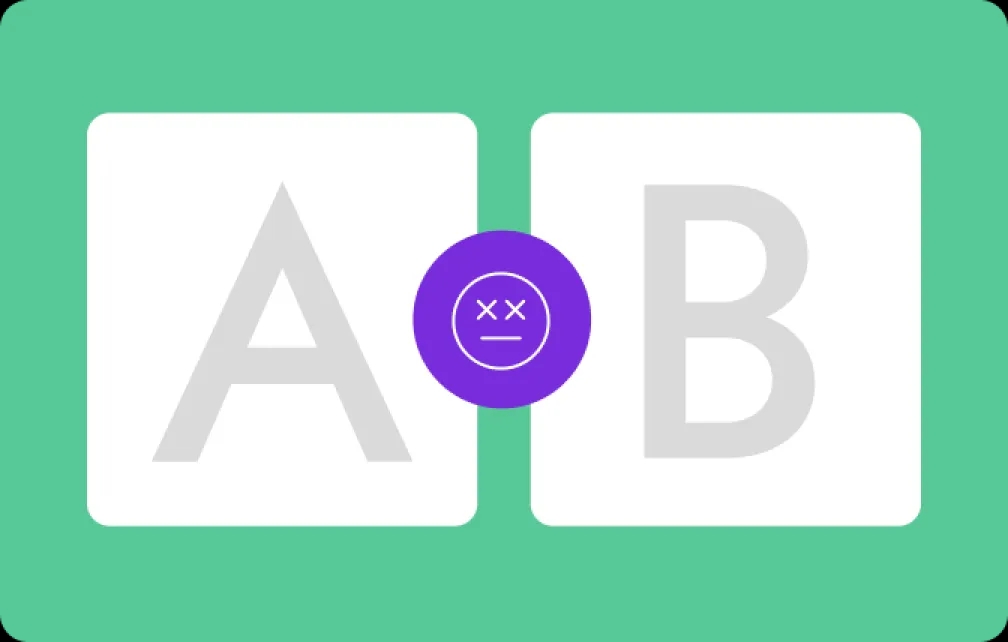
Lifecycle marketers know that when it comes to engaging their customers, practice beats theory. No matter how brilliant a campaign, email subject, ad, or creative seems on paper, the only way to know if an idea works is to try it out with customers. Traditionally, marketers have tried out their ideas through A/B testing.
But as many marketers have learned, A/B testing has problems.
Problem #1: A/B testing is slow
To set up an A/B test, marketers first need to divide their customers into segments. Then they must run tests on each segment, wait for data to come in, and then analyze and implement the results. These steps take time, but markets move quickly. Results from a test run at one time, or with one segment, might not make good predictions as markets and demographics change. The results of the A/B test are always a trailing indicator.
Problem #2: A/B testing doesn’t scale
Manual testing can seem feasible if you only have one variable to test – for example, which of two logos is more appealing to your customers? But a typical marketing campaign requires dozens of choices. Should we use paid ads or our owned channels? Should we reach customers via phone, email, or SMS? How often should we send messages? What time should we send them? You could try to test each of these variables for each segment. But you will quickly reach a “combinatorial explosion” – there are simply too many variables to test.
All too often, marketers faced with these challenges will tell themselves, “well, maybe it’s okay if we don’t test everything. Let’s just send all the emails at 3 pm.” Of course, going with your best guess often means a less effective campaign.
Problem #3: A/B testing offers personalization that isn’t very personal
When an A/B test comes back with a clear result, it feels like a win. “Subject line A got more opens than subject line B!” The problem is that some customers connected better with subject line B. If marketers adopt the “winning” option from the A/B test, they are simply letting the majority rule. In an election, getting 51% of the vote means you win. In a marketing campaign, engaging 51% of your customers is probably a losing strategy – 49% of your customers aren’t getting what they want.
What about segments? Dividing customers into segments is better than nothing, but it doesn’t solve the problem. You are still deciding how to engage each customer based on a single data point – their segment. Today’s lifecycle marketers have rich first-party data moldering in their data warehouses and CDPs. A/B testing by segment lets the depth of that data go to waste.
The solution: AI decisioning
Savvy marketers have begun using a new application of machine learning, called reinforcement learning (RL), or AI decisioning, to replace A/B testing. At OfferFit, we provide an AI Decisioning Engine that lifecycle marketers use for 1:1 personalization.
To get started, marketers make three choices.
Success metric. What is the KPI you want to maximize?
Experimentation dimensions. What are the dimensions along which you’d like to test? (e.g., offer, subject line, creative, channel, timing, cadence, etc.)
Options. What are the options available for each dimension?
From there, the AI decisioning agents experiment and personalize. An engine like OfferFit's makes daily recommendations for each customer, seeking to maximize the chosen success metric. The AI decisioning agent learns from every customer interaction, and applies these insights to the next day's recommendations.
AI decisioning can be a powerful tool to help markers test at scale. But the experiments carried out by an AI agent are not simply manual experiments done more quickly. The advantages over manual testing are fundamental.
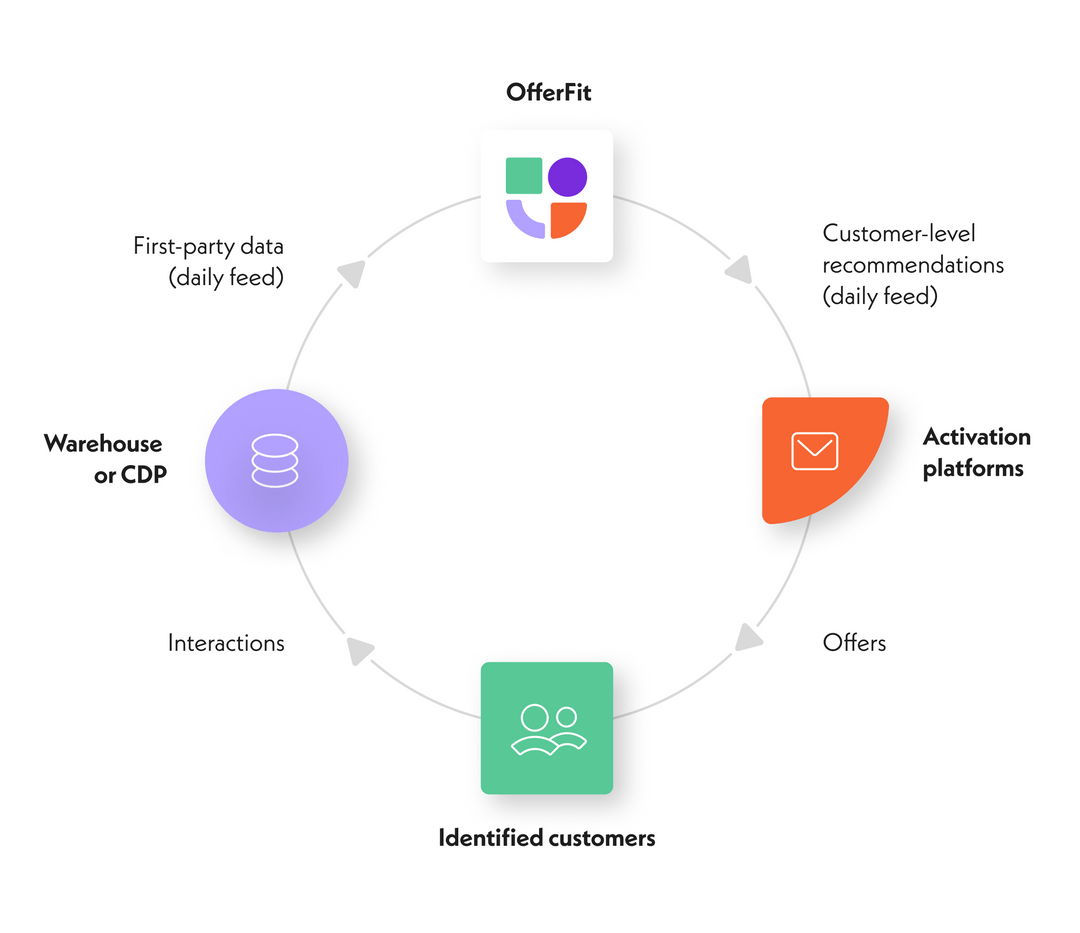
AI decisioning is fast and flexible
AI decisioning is faster and easier than manual A/B testing. There is no need to set up segments, design experiments, analyze the data, and implement the results. Instead, the process is automated, and the AI agent continuously learns and improves.
AI decisioning provides continuous learning
There’s no need to administer new tests when conditions change – AI decisioning provides continuous learning. A traditional A/B test is static, giving a snapshot at a moment in time. If marketers want new insights as market conditions change, they must set up and administer new tests. Unlike a traditional A/B test, a self-learning agent constantly learns and adapts as the market changes.
AI decisioning provides 1:1 personalization
With manual A/B testing, marketers are stuck in a “majority rules” regime, giving each customer the most popular option for their segment. A traditional A/B test concludes by saying, “70% of customers in segment X preferred option Y.” A customer is reduced to a single data point – the segment.
AI decisioning agents make the best decision for each customer individually, making customer segments obsolete. The “winning” option for a particular customer at a specific time depends on all the data the AI agent has at the moment it makes the decision.
Ready to learn more about AI testing? Download our whitepaper or schedule a demo with one of our experts.
Ready to start personalizing with AI decisioning?